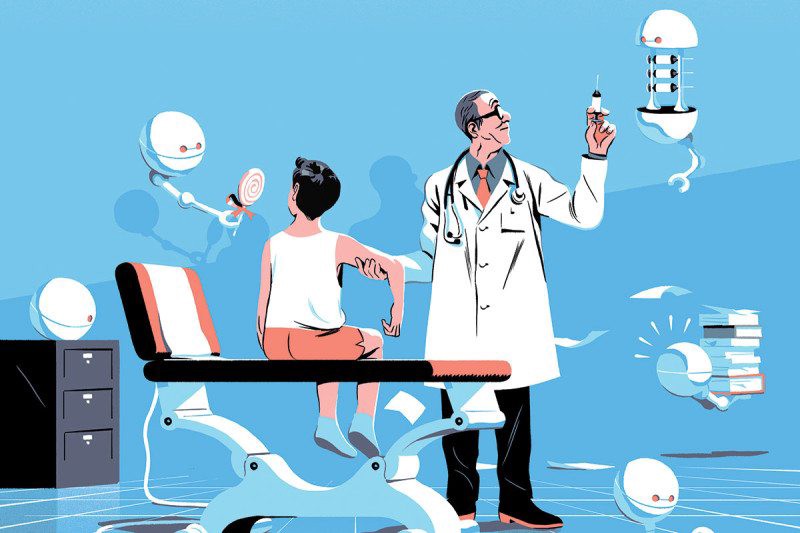
AI-Based Tools Predict COVID-19 Disease Severity
Two healthcare workers under the age of 30 fell ill in Wuhan, China, where the first COVID-19 case was reported. One survived. The other was not as fortunate. But why?
It is an example researchers at the Radiological Society of North America highlighted while pointing out that this phenomenon—some patients falling critically ill and dying as others experience minimal symptoms or none at all—is one of the most mysterious elements of this disease. Mortality does correlate with factors such as age, gender, and some chronic conditions. Considering young and previously healthy individuals have succumbed to this virus, though, there could be more complex prognostic factors involved.
Current diagnostic tests determine whether or not individuals have the virus. They do not, however, offer clues as to just how sick a COVID-positive patient could become. For the time being, clinicians cannot easily predict which patients who test positive will require hospital admission for oxygen and possible ventilation.
Because most cases are mild, identifying those at risk for severe and critical cases early on could help healthcare facilities prioritize care and resources such as ventilators and ICU beds. Figuring out who is at low risk for complications could be useful, too, as this could reduce hospital admissions while these patients are managed at home. As health systems across the globe continue to deal with large numbers of COVID-19 cases, new and emerging technologies may be able to help in this regard.
AI Plus Imaging
Researchers have been probing he use of AI and imaging to determine who has COVID-19, but some groups are taking a different approach and using this same combination to determine which patients are most likely to need the most extensive treatment.
In a paper published on July 22 in Radiology: Artificial Intelligence, researchers at Massachusetts General Hospital and Harvard Medical School reveal efforts to develop an automated measure of COVID-19 pulmonary disease severity using chest radiographs (CXRs) and a deep-learning algorithm.
Elsewhere, an international group proposed an AI model that uses COVID-19 patient"s geographical, travel, health, and demographic data to predict disease severity and outcome. Future work is expected to focus on the development of a pipeline that combines CXR scanning models with these types of healthcare data and demographic processing models, according to their paper published July 3 in Frontiers in Public Health.
In June, GE Healthcare announced a partnership with the University of Oxford-led National Consortium of Intelligent Medical Imaging (NCIMI) in the UK to develop algorithms aimed at predicting COVID-19 severity, complications, and long-term impact.
Similarly, experts at the University of Copenhagen set out to create models that calculate the risk of a COVID-19 patient"s need for intensive care. The algorithms are designed to find patterns among Danish coronavirus patients who have been through the system to find shared traits among the most severely affected. The patterns are compared with data gathered from recently hospitalized patients, such as X-rays, and sent to a supercomputer to predict how likely a patient is to require a ventilator and how many days will pass before that need arises.
Meanwhile, researchers at Case Western Reserve University are using computers to find details in digital images of chest scans that are not easily seen by the human eye to quickly determine which patients are most likely to experience further deterioration of their health and require the use of ventilators.
“The approach we have taken is actually to create a synergistic artificial intelligence algorithm—one that combines patterns from CT scans with clinical parameters based on lab values,” Anant Madabhushi, professor of biomedical engineering at Case Western Reserve and head of the Center for Computational Imaging and Personalized Diagnostics (CCIPD) told Diagnostics World.
“And the secret sauce, if you will, is the fact that we are using neural networks and deep learning to automatically go into the CT scans and identify exactly where the region of disease is,” he added. Zeroing in on the disease presentation on the CT scan makes it possible to mine patterns using the neural networks from those regions and combine them with the clinical parameters.
Madabhushi and colleagues have completed a multi-site study that included nearly 900 patients from Wuhan, China, and Cleveland, Ohio. They found that the combination of the clinical parameters and imaging features yielded a higher predictive accuracy in identifying who would go on to need a ventilator compared to a model that uses the imaging features alone and also compared to a model that used only the clinical parameters.
The inspiration for this work came about months ago as Italy hit its peak and the country"s hospitals were overwhelmed with patients who could not breathe. Some of the stories were gut-wrenching, he explained, particularly the ones that highlighted how physicians had to make a case by case determinations about who got a ventilator and who did not.
“It really got me thinking about what the implications are for the US or the rest of the world,” he said if a second wave materializes in the fall as some experts have predicted. Of course, we are not out of the first wave yet, he acknowledged, but there is a real concern that a second wave could be even deadlier than the first considering it would take place during flu season.
Madabhushi and colleagues began building their model using images and datasets found online in early March. In April, the CCIPD was offered digital images of chest scans taken from roughly 100 early victims of the novel coronavirus from Wuhan, China. Using that information, the researchers developed machine learning models to predict the risk of a COVID-19 patient needing a ventilator—one based on neural networks and another derived from radionics.
Early CT scans from patients with COVID-19 showed distinctive patterns specific to those in the intensive care unit (ICU) compared to those, not in the ICU. Initially, the research team was able to achieve an accuracy of roughly 70% to 75%. Since then, they have improved upon that performance metric, he said, raising the accuracy level to about 84%.
They have worked to circumvent bias by exposing the AI to patients from different demographics, ethnicities, populations, and scanners. But there is still work to be done, including additional multi-site testing and prospective field testing. Madabhushi hopes to validate the technology on patients from the Louis Stokes Cleveland VA Medical Center, where he is a research scientist and is looking to prove the technology at Cleveland Clinic as well.
The team is also developing a user interface that couples the AI with a tool that allows the end-user to enter a CT scan and clinical parameters to see the likelihood of needing a ventilator. Before clinically deploying the technology, he wants to put this in the hands of end-users for additional prospective field testing so that users can get comfortable with the tool, get a sense of how to work with it, and learn how to interpret and use the results coming out of it.
Rather than making arbitrary decisions about who gets a ventilator and who does not, the big hope is that this type of triaging technology could enable more rational decision-making for appropriate resources.
AI and Blood Biomarkers
Another group was also motivated by the scenario that played out in northern Italy back in February and March as a lack of ICU beds led to tough decisions for clinicians.
“Unfortunately, this process, I would say, is a little bit cyclical,” John T. McDevitt, professor of biomaterials at NYU College of Dentistry and professor of chemical and molecular engineering at NYU Tandon School of Engineering told Diagnostics World. Similar scenarios have played out in New York City, for instance, and more recently in Houston. “When you hit this point where you do not have any buffer, any excess capacity, then it forces a very difficult situation.”
He wants to provide clinicians with what he describes as “a flashlight that goes into this dark room of COVID-19 severity.” The intent is to look into the future and attempt to figure out which patients will perish unless extreme measures are taken, which patients should be admitted to the hospital, and which patients can safely recover from home.
“I would describe this as the third leg of the stool for the diagnosis and prognosis of COVID-19,” he explained. PCR testing has been used to determine whether individuals have the disease, and serology testing has helped establish whether people have had the condition in the past. The missing leg here, he said, has been determining which patients are going to end up in the hospital and which patients are most likely to perish.
To fill that void, he and colleagues have developed a smartphone app that uses AI and biomarkers inpatient"s blood to determine COVID-19 disease severity. Their findings were published June 3 in Lab on a Chip.
Relying on data from 160 hospitalized COVID-19 patients in Wuhan, China, they found four biomarkers measured in blood tests that were elevated in the patients who died compared with those who recovered. These biomarkers (C-reactive protein, myoglobin, procalcitonin, and cardiac troponin I) can signal complications relevant to COVID-19, such as reduced cardiovascular health, acute inflammation, or lower respiratory tract infection.
The researchers then developed a model using the biomarkers as well as age and sex—two risk factors. They trained the model to define the patterns of COVID-19 disease and predict its severity. When a patient"s information is entered, the model comes up with a numerical severity score ranging from 0 (mild) to 100 (critical), reflecting the probability of death from the complications of COVID-19.
It was validated using information from 12 hospitalized COVID-19 patients from Shenzhen, China, and further validated using data from over 1,000 New York City patients. The app has also been evaluated in the Family Health Centers at NYU Langone in Brooklyn.
The diagnostic system uses small samples, such as swabs of saliva or drops of blood from a fingertip, which are added to credit card-sized cartridges. The cartridge is put into a portable analyzer that tests for a range of biomarkers, with results available in under 30 minutes. After optimizing the app"s clinical utility, the goal is to roll it out nationwide and worldwide.
Over the coming months, McDevitt"s laboratory, in partnership with SensoDx—a company spun out of his lab—intends to develop and scale the ability to produce a severity score similar to the way people with diabetes check their blood sugar. The plan is to distribute the tool first to disease epicenters to maximize its impact considering not all locations are dealing with a shortage of ICU beds or respirators.
McDevitt also highlighted the potential to help address racial disparities. “COVID has ripped the scab off of this particular wound,” he said. This technology can help level the healthcare playing field and remove some of the unintentional racial or ethnic biases that may weave their way into the delivery of healthcare. By putting the severity score on a numerical index, it arguably provides a more objective way to make challenging pandemic-related healthcare decisions.
McDevitt and colleagues are not the only ones pursuing blood-based biomarkers for the prediction of COVID-19 disease severity.
Another example can be found in a study published May 14 in Nature Machine Intelligence and conducted by a group of Chinese researchers, who used a database of blood samples from nearly 500 infected patients in the Wuhan region.
Their machine learning-based model predicts the mortality rates of patients over 10 days in advance with more than 90% accuracy, according to the paper, using three biomarkers: lactic dehydrogenase, lymphocyte, and high-sensitivity C-reactive protein.